Statistical Significance in Marketing: Q&A with SeQuel’s Senior Marketing Strategist, Preston Carroll
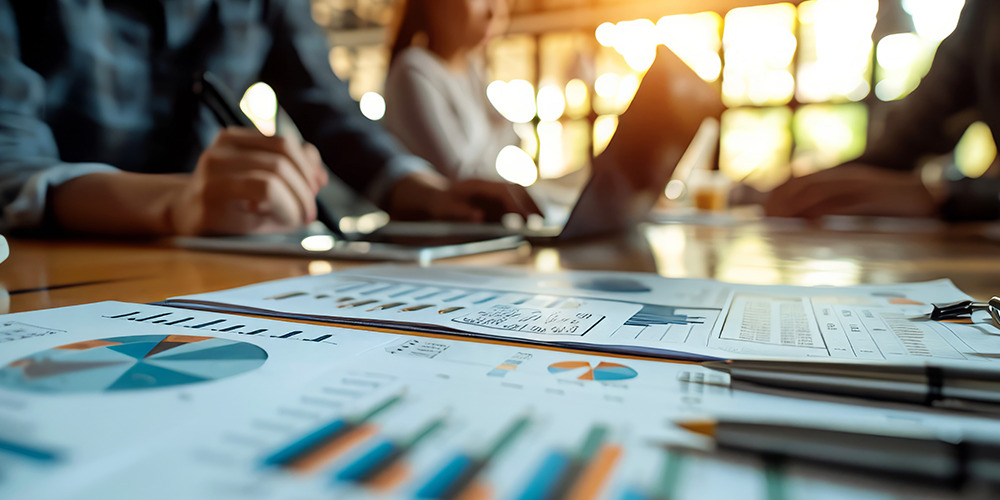
Measuring statistical significance in marketing is essential for making data-driven decisions. It allows marketers to assess the effectiveness of marketing campaigns, understand the impact of various strategies, and identify patterns or relationships in performance data.
We sat down with SeQuel’s Senior Marketing Strategist, Preston Carroll, to answer your questions about statistical significance in marketing and how to use this type of analysis to draw actionable insights into campaign performance.
What is statistical significance?
Carroll: Statistical significance (stat sig) helps to determine if the results of a test are statistically meaningful or if they could have occurred by chance alone.
Why is statistical significance important in marketing campaigns?
Carroll: Not all changes in metrics during a test campaign (such as conversion rates in a mail campaign) are meaningful. Some might be due to random chance, rendering the test inconclusive. By establishing statistical significance, marketers can be more confident that the changes observed are real and can influence future strategies (creative or targeting) or help identify trends that can optimize a program.
How do I make sure a test is set up for statistical significance?
Carroll: When running a test for statistical significance in direct mail, you’ll want to make sure you follow these principles:
- Determine the Control Group
- Hold All Else Equal
- Assign a Significant Test Quantity
- Determine if the Test Result will be Significant
Principle 1: Determine the Control Group
If you are going to test a new List, you will want to identify what list you will be testing against. Often this is referred to as the “Control”, or “Baseline” group. Moreover, the Control group is typically your best performer at the time of the test.
Principle 2: Hold All Else Equal
Once you’ve determined what you’re testing, and what you’re testing against (Control), you’ll want to make sure that every other variable is held constant across Test and Control groups. In this way, you’re setting up an AB test environment. For example, if you’re testing a new list, you’ll want to make sure that the Control list and Test List have the same creative, offer and timing so you can measure the main effects of the test. More scientifically, your test hypothesis is that the new list will positively affect conversion rates, so you want to make sure that any difference in performance between the test and control groups is determined by the difference in the list.
Principle 3: Assign a Significant Test Quantity
Statistical significance is used in determining both the required test sample size and if the result of the test is meaningful (see Principal 4).
To determine the required test sample size for statistical significance, you will want to have an estimated response rate, an allowable margin of error and a confidence level goal.
- The estimated response rate can be influenced by your Control mail group, or an educated guess (for those who have not mailed before).
- The margin of error is a range of uncertainty surrounding that estimated response rate (this is typically 10%, but can be influenced by previous marketing!).
- The confidence level is a product of the aforementioned and tells you how confident you can be that the sample size (and subsequent result) is significant. Confidence level goals tend to range from 80% to 99%, depending on the preference of the marketer.
For example, if we assume a response rate of 0.5%, a margin of error at 10% and a goal confidence level of 90%, you will need to mail ~50,000 pieces at a minimum for this sample to be categorized as statistically significant. Please contact a SeQuel strategist to determine test cell sizes for your unique program.
Ultimately, the significance of a test sample greatly depends on the estimated response rate. There’s an inverse relationship between response rate and required quantity for stat sig (e.g., high response rate = lower quantity).
Principle 4: Determine if the Test Result will be Significant
When you put the test in market and read performance, you will compare the sample size and response rates for the Control and Test groups to see if the difference in response rates is statistically significant, or inconclusive.
Analyzing if a result is statistically significant is purely based on the difference in performance across control and test groups, either positive or negative. So, if you see the test win or lose by X%, a stat sig calculation will tell if that difference (“X%”) is significant or not at a given confidence level, (e.g., the difference is real rather than happenstance).
How do I determine the ideal level of confidence for my test?
Carroll: Industry standards suggest a stat sig confidence level of 80% to 99% as a common threshold. Confidence levels may vary based on campaign objectives, resources, and target audience. A higher level of confidence implies greater level of statistical significance in the result, but may require larger sample sizes, longer campaign durations and a higher financial investment.
What are some challenges I may experience when trying to achieve statistical significance?
Carroll: The size of your data sample can greatly affect the ability to detect statistically significant results. Smaller samples may not provide enough data to reveal true effect, but some marketers may not have the budgets required to get a large enough sample to achieve statistical significance. Another potential challenge is the response rate deltas between the control and test groups, based on the sample sizes. For large samples sizes in control and test groups, the test group will need to perform X% better than the control group to achieve statistical significance. For small sample sizes in control and test groups, the test group will need to perform X+Y% better than the control group to achieve statistical significance. In other words, even if you have a stat sig sample size in the test group, the test may not perform different enough to warrant a stat sig result (compared to control).
______________________
While statistical significance provides mathematical confidence in your results, it should be paired with practical significance and a solid understanding of your market to inform strategic decisions.
If you’re ready to differentiate between real effects and random noise, optimize your program performance, and ultimately drive better business outcomes, contact a SeQuel Marketing Strategist today.